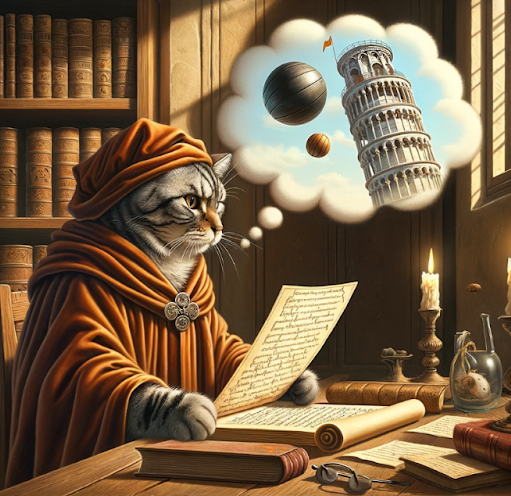
Do Androids Dream? World Models in Modern AI
One of the most striking AI advances this spring...
Facial identification and verification for consumer and security applications.
Activity recognition and threat detection across camera views.
Spatial computing, gesture recognition, and gaze estimation for headsets.
Millions of identities and clothing options to train best-in-class models.
Simulate driver and occupant behavior captured with multi-modal cameras.
Simulate edge cases and rare events to ensure the robust performance of autonomous vehicles.
Together, we’re building the future of computer vision & machine learning
Facial identification and verification for consumer and security applications.
Activity recognition and threat detection across camera views.
Spatial computing, gesture recognition, and gaze estimation for headsets.
Millions of identities and clothing options to train best-in-class models.
Simulate driver and occupant behavior captured with multi-modal cameras.
Simulate edge cases and rare events to ensure the robust performance of autonomous vehicles.
Together, we’re building the future of computer vision & machine learning
Facial identification and verification for consumer and security applications.
Activity recognition and threat detection across camera views.
Spatial computing, gesture recognition, and gaze estimation for headsets.
Millions of identities and clothing options to train best-in-class models.
Simulate driver and occupant behavior captured with multi-modal cameras.
Simulate edge cases and rare events to ensure the robust performance of autonomous vehicles.
Together, we’re building the future of computer vision & machine learning
Facial identification and verification for consumer and security applications.
Activity recognition and threat detection across camera views.
Spatial computing, gesture recognition, and gaze estimation for headsets.
Millions of identities and clothing options to train best-in-class models.
Simulate driver and occupant behavior captured with multi-modal cameras.
Simulate edge cases and rare events to ensure the robust performance of autonomous vehicles.
Together, we’re building the future of computer vision & machine learning
One of the most striking AI advances this spring was OpenAI’s Sora, a video generation model that sets new standards for video consistency and diversity. Interestingly, the official report on Sora is titled “Video generation models as world simulators”. It notes that Sora has emerging simulation capabilities and is on a “promising path towards the development of capable simulators of the physical and digital world”. Today, we discuss world models in modern artificial intelligence: what they are, how they have progressed over the last few years, and where they may go in the future.
One of the most striking AI advances this spring was OpenAI's Sora, a video generation model that sets new standards…
The announcement of Gemini 1.5 by Google was all but eclipsed by OpenAI’s video generation model Sora. Still, there was…
One of the most interesting AI-related news for me recently was a paper by DeepMind researchers that presented a new…
Here at Synthesis AI, we have decided to release the "Generative AI" series in an e-book form; expect a full-fledged…
This is the last post in the "Generative AI" series. Today, we look into the future and discuss where the…
Last time, we finished all intended mathematical content, so it is time for us to wrap up the generative AI…
CVPR 2022, the largest and most prestigious conference in computer vision and one of the most important ML venues in general, has just finished in New Orleans. With over 2000 accepted papers, reviewing the contributions of this year’s CVPR appears to be a truly gargantuan task. Over the next series of blog posts, we will attempt to go over the most interesting papers directly related to our main topic: synthetic data. Today, I present the first but definitely not the last installment devoted to papers from CVPR 2022.
Last time, we started a new series of posts: an overview of papers from CVPR 2022 that are related to synthetic data. This year’s CVPR has over 2000 accepted papers, and many of them touch upon our main topic on this blog. In today’s installment, we look at papers that make use of synthetic data to advance a number of different use cases in computer vision, along with a couple of very interesting and novel ideas that extend the applicability of synthetic data in new directions. We will even see some fractals as synthetic data! (image source)
Last time, we talked about new use cases for synthetic data, from crowd counting to fractal-based synthetic images for pretraining large models. But there is a large set of use cases that we did not talk about, united by their relation to digital humans: human avatars, virtual try-on for clothes, machine learning for improving animations in synthetic humans, and much more. Today, we talk about the human side of CVPR 2022, considering two primary applications: conditional generation for applications such as virtual try-on and learning 3D avatars from 2D images (image generated by DALL-E-Mini by craiyon.com with the prompt “virtual human in the metaverse”).
We continue the long series of reviews for CVPR 2022 papers related to synthetic data. We’ve had three installments so far, devoted to new datasets, use cases for synthetic data, and a very special use case: digital humans. Today, we will discuss papers that can help with generating synthetic data, so expect a lot of 3D model reconstruction, new generative models, especially in 3D, and generally a lot of CGI-related goodness (image generated by DALL-E-Mini by craiyon.com with the prompt “robot designer making a 3D mesh”).
Today, we are kicking off the Synthesis AI blog. In these posts, we will speak mostly about our main focus, synthetic data, that is, artificially created data used to train machine learning models. But before we begin to dive into the details of synthetic data generation and use, I want to start with the problem setting. Why do we need synthetic data? What is the problem and are there other ways to solve it? This is exactly what we will discuss in the first series of posts.
In the previous post, we posed what we consider the main problem of modern machine learning: increasing appetite for data that cannot be realistically satisfied if current trends persist. This means that current trends will not persist — but what is going to replace them? How can we build machine learning systems at ever increasing scale without increasing the need for huge hand-labeled datasets? Today, we consider one possible answer to this question: one-shot and zero-shot learning.
Today, we continue our series on the data problem in machine learning. In the first post, we realized that we are already pushing the boundaries of possible labeled datasets. In the second post, we discussed one way to avoid huge labeling costs: using one-shot and zero-shot learning. Now we are in for a quick overview of the kind of machine learning that might go without data at all: reinforcement learning.
In the first three posts of this series, we have seen several ways to overcome the data problem in machine learning: first we posed the problem, then discussed one-shot and zero shot learning, and in the third post presented the reinforcement learning way of using no data at all. In this final installment, we discuss the third direction that modern machine learning takes to help with the lack of labeled data: how can we use unlabeled data to help inform machine learning models?
Inside AI Ask Me Anything (AMA) with CEO and Founder, Yashar Behzadi
Synthesis AI CEO and Founder Yashar Behzadi recently sat down for an Ask Me Anything with our friends at Inside AI. The discussion was wide-ranging, touching on what’s next for generative AI, how synthetic data is generated and used, overcoming model bias, and the ethics of AI systems. If you’re tinkering with generative AI and need representative synthetic human data for developing ML models ethically, we’d love to talk — contact us any time.
Previously on this blog, we have discussed the data problem: why machine learning may be hitting a wall, how one-shot and zero-shot learning can help, how come reinforcement learning does not need data at all, and how unlabeled datasets can inform even supervised learning tasks. Today, we begin discussing our main topic: synthetic data. Let us start from the very beginning: how synthetic data was done in the early days of computer vision…
We continue from last time, when we began a discussion of the origins and first applications of synthetic data: using simple artificial drawings for specific problems and using synthetically generated datasets to compare different computer vision algorithms. Today, we will learn how people made self-driving cars in the 1980s and see that as soon as computer vision started tackling real world problems with machine learning, it could not avoid synthetic data.
Optical 3D range sensors, like RGB-D cameras and LIDAR, have found widespread use in robotics to generate rich and accurate 3D maps of the environment, from self-driving cars to autonomous manipulators. However, despite the ubiquity of these complex robotic systems, transparent objects (like a glass container) can confound even a suite of expensive sensors that are commonly used.
Synthetic Data for Robots, Part I: Are Simulations Good For Robotics?
In the previous two blog posts, we have discussed the origins and first applications of synthetic data. The first part showed how early computer vision used simple line drawings for scene understanding algorithms and how synthetic datasets were necessary as test sets to compare different computer vision algorithms. In the second part, we saw how self-driving cars were made in the 1980s and how the very first application of machine learning in computer vision for autonomous vehicles, the ALVINN system, was trained on synthetic data. Today, we begin the discussion of early robotics and the corresponding synthetic simulators… but this first part will be a bit more philosophical than usual.
Synthetic Data for Early Robots, Part II: MOBOT and the Problems of Simulation
Last time, we talked about robotic simulations in general: what they are and why they are inevitable for robotics based on machine learning. We even touched upon some of the more philosophical implications of simulations in robotics, discussing early concerns on whether simulations are indeed useful or may become a dead end for the field. Today, we will see the next steps of robotic simulations, showing how they progressed after the last post with the example of MOBOT, a project developed in the first half of the 1990s in the University of Kaiserslautern. This is another relatively long read and the last post in the “History of Synthetic Data” series.
Object Detection with Synthetic Data I: Introduction to Object Detection
Today, we begin a new mini-series that marks a slight change in the direction of the series. Previously, we have talked about the history of synthetic data (one, two, three, four) and reviewed a recent paper on synthetic data. This time, we begin a series devoted to a specific machine learning problem that is often supplemented by the use of synthetic data: object detection. In this first post of the series, we will discuss what the problem is and where the data for object detection comes from and how you can get your network to detect bounding boxes like below (image source).
Object Detection with Synthetic Data II: Common Objects And Their Context
In the last post, we started talking about object detection. We discussed what the problem is, saw the three main general-purpose real-world datasets for object detection, and began talking about synthetic data. Today, we continue the series with a brief overview of the most important synthetic datasets for object detection. Last time, I made an example of an autonomous driving dataset, but this is a topic of its own, and so are, say, synthetic images of people and human faces. Today, we will concentrate on general-purpose and household object datasets.
Object Detection with Synthetic Data III: Choose Your Cues Wisely
Today, I continue the series on synthetic data for object detection. In the first post of the series, we discussed the object detection problem itself and real world datasets for it, and the second was devoted to popular synthetic datasets of common objects. The time has come to put this data in practice: in this and subsequent posts, we will discuss common contemporary object detection architectures and see how adding synthetic data fares for object detection as reported in literature. In each post, I will give a detailed account of one paper that stands out in my opinion and briefly review one or two more. We begin in 2015.
Object Detection with Synthetic Data IV: What’s in the Fridge?
We continue the series on synthetic data for object detection. Last time, we stopped in 2016, with some early works on synthetic data for deep learning that still have implications relevant today. This time, we look at a couple of more recent papers devoted to multiple object detection for food and small vendor items. As we will see today, such objects are a natural application for synthetic data, and we’ll see how this application has evolved in the last few years.
Object Detection with Synthetic Data V: Where Do We Stand Now?
This is the last post in my mini-series on object detection with synthetic data. Over the first four posts, we introduced the problem, discussed some classical synthetic datasets for object detection, talked about some early works that have still relevant conclusions and continued with a case study on retail and food object detection. Today we consider two papers from 2019 that still represent the state of the art in object detection with synthetic data and are often used as generic references to the main tradeoffs inherent in using synthetic data. We will see and discuss those tradeoffs too. Is synthetic data ready for production and how does it compare with real in object detection? Let’s find out. (header image source)
Driving Model Performance with Synthetic Data I: Augmentations in Computer Vision
Welcome back, everybody! It’s been a while since I finished the last series on object detection with synthetic data (here is the series in case you missed it: part 1, part 2, part 3, part 4, part 5). So it is high time to start a new series. Over the next several posts, we will discuss how synthetic data and similar techniques can drive model performance and improve the results. We will mostly be talking about computer vision tasks. We begin this series with an explanation of data augmentation in computer vision; today we will talk about simple “classical” augmentations, and next time we will turn to some of the more interesting stuff.
Smart Augmentations: Driving Model Performance with Synthetic Data II
Last time, I started a new series of posts, devoted to different ways of improving model performance with synthetic data. In the first post of the series, we discussed probably the simplest and most widely used way to generate synthetic data: geometric and color data augmentation applied to real training data. Today, we take the idea of data augmentation much further. We will discuss several different ways to construct “smart augmentations” that make much more involved transformations of the input but still change the labeling only in predictable ways.
Domain Adaptation Overview: Driving Model Performance with Synthetic Data III
Today, I continue the series about different ways of improving model performance with synthetic data. We have already discussed simple augmentations in the first post and “smart” augmentations that make more complex transformations of the input in the second. Today we go on to the next sub-topic: domain adaptation. We will stay with domain adaptation for a while, and in the first post on this topic I would like to present a general overview of the field and introduce the most basic approaches to domain adaptation.
Gaze Estimation and GANs: Driving Model Performance with Synthetic Data IV
With the Christmas and New Year holidays behind us, let’s continue our series on how to improve the performance of machine learning models with synthetic data. Last time, I gave a brief introduction into domain adaptation, distinguishing between its two main variations: refinement, where synthetic images are themselves changed before they are fed into model training, and model-based domain adaptation, where the training process changes to adapt to training on different domains. Today, we begin with refinement for the same special case of eye gaze estimation that kickstarted synthetic data refinement a few years ago and still remains an important success story for this approach, but then continue and extend the story of refinement to other computer vision problems. Today’s post will be more in-depth than before, so buckle up and get ready for some GANs!
Synthetic-to-Real Refinement: Driving Model Performance with Synthetic Data V
We continue the series on synthetic data as it is used in machine learning today. This is a fifth part of an already pretty long series (part 1, part 2, part 3, part 4), and it’s far from over, but I try to keep each post more or less self-contained. Today, however, we pick up from last time, so if you have not read Part 4 yet I suggest to go through it first. In that post, we discussed synthetic-to-real refinement for gaze estimation, which suddenly taught us a lot about modern GAN-based architectures. But eye gaze still remains a relatively small and not very variable problem, so let’s see how well synthetic data does in other computer vision applications. Again, expect a lot of GANs and at least a few formulas for the loss functions.
Real-to-Synthetic Data: Driving Model Performance with Synthetic Data VI
Today we continue the series on using synthetic data to improve machine learning models.This is the sixth part of the series (Part I, Part II, Part III, Part IV, Part V). In this (relatively) short interlude I will discuss an interesting variation of GAN-based refinement: making synthetic data from real. Why would we ever want to do that if the final goal is always to make the model work on real data rather than synthetic? In this post, we will see two examples from different domains that show both why and how.
Driving Model Performance with Synthetic Data VII: Model-Based Domain Adaptation
After a long hiatus, we return from interviews to long forms, continuing (and hopefully finishing) our series on how synthetic data is used in machine learning and how machine learning models can adapt to using synthetic data. This is our seventh installment in the series (part 1, part 2, part 3, part 4, part 5, part 6), but, as usual, this post is (I hope!) sufficiently self-contained. We will discuss how one can have a model that works well on synthetic data without making it more realistic explicitly but doing the domain adaptation work at the level of features or model itself.