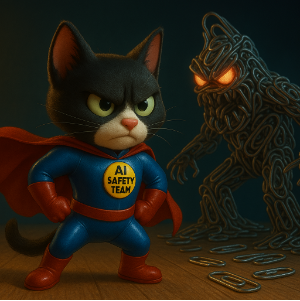
- Applications
Biometrics & security
ID verification
Facial identification and verification for consumer and security applications.
Security
Activity recognition and threat detection across camera views.
Consumer devices & applications
AR/VR/XR
Spatial computing, gesture recognition, and gaze estimation for headsets.
Virtual try-on
Millions of identities and clothing options to train best-in-class models.
Biometrics & security
Driver monitoring
Simulate driver and occupant behavior captured with multi-modal cameras.
Pedestrian detection
Simulate edge cases and rare events to ensure the robust performance of autonomous vehicles.
- Resources
- Company
Join Our Team
Together, we’re building the future of computer vision & machine learning
- Applications
Biometrics & security
ID verification
Facial identification and verification for consumer and security applications.
Security
Activity recognition and threat detection across camera views.
Consumer devices & applications
AR/VR/XR
Spatial computing, gesture recognition, and gaze estimation for headsets.
Virtual try-on
Millions of identities and clothing options to train best-in-class models.
Biometrics & security
Driver monitoring
Simulate driver and occupant behavior captured with multi-modal cameras.
Pedestrian detection
Simulate edge cases and rare events to ensure the robust performance of autonomous vehicles.
- Resources
- Company
Join Our Team
Together, we’re building the future of computer vision & machine learning