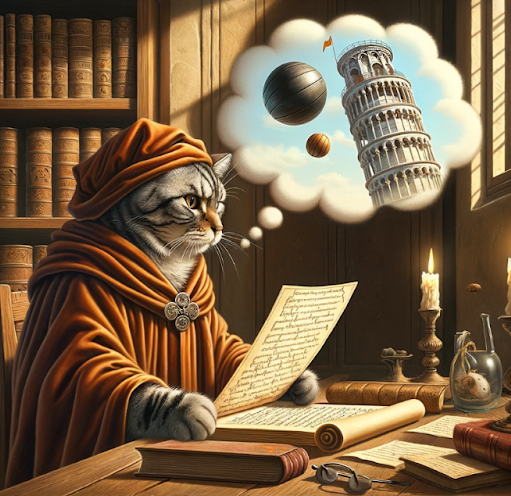
Do Androids Dream? World Models in Modern AI
One of the most striking AI advances this spring...
This is the last post in the “Generative AI” series. Today, we look into the future and discuss where the current trends take us, what dangers might artificial general intelligence (AGI) hold for us, and whether we are ready for these dangers (spoiler: not at all). I will present the case for AGI doomers and discuss the main arguments, but please keep in mind that in this post, everything is mostly speculation (although there actually are attempts to put this speculation on firm mathematical ground).
We ended the last post on the differences between slow and fast takeoff speeds in AI development. But regardless of whether superhuman AGI comes overnight or several years after reaching approximately human level, it still may come pretty quickly even with respect to human timescales. We better be ready to face AGI in our lifetimes. Are we?
The previous post read as a glowing review of the latest developments, and that was intentional. In this post, let me posit right off the bat that the rise of large language models is worrying as much as it is exhilarating. Here is our plan for today, with a rough classification of different levels of potential risks related to human-level and ultimately superhuman intelligence:
We will begin with what AI researchers usually call “mundane problems”. These are the problems you already hear about on the news sometimes: modern large language models can be jailbroken and then start giving away dangerous information or insult users, modern image generation models can be used to create convincing deepfakes, AI models have biases that come either from the training data or the model architecture itself, and so on. These problems are not entirely new, but I’m positive we can either resolve them or at least become accustomed to them.
As AI becomes a larger part of the economy (which it almost certainly will), the risks grow as well. Even without reaching superhuman levels, AI is already a transformative technology, leading to a kind of new industrial revolution where many previous jobs may become obsolete. So far, transformations like this have been tumultuous but always ultimately positive: they have always created more and better jobs than they destroyed. Will this be the case for AI as well?
Finally, even the economy takes a back seat compared to existential risks. We humans are quite new to the idea: true, we have had nuclear weapons able to eliminate humanity (although not really), and the climate change may at some point approach an existential risk, but AI-related risks may prove to be very different, and we will discuss why.
We will end this last post with a brief overview of what people are currently doing about these risks through the emerging field of AI alignment research. In brief, we hope this research will arrive on time to save us all, but we are still far from a solution.
The “mundane problems” are those you hear about when GPT-4 makes the news: AI posing as a human, deepfakes fooling real people with images or voice, and so on. We will see that AI-related dangers are far from limited to the mundane, but let us first consider those.
First, jailbreaking: the art of making a large language model disobey its explicit instructions (that have been fine-tuned into the model by its developers, probably by RLHF or similar techniques that we discussed the previous post) and exhibit some kind of antisocial behavior. All large language models that we discussed have been jailbroken in some way. You cannot rely on RLHF or other fine-tuning approaches if you are dealing with a determined adversary, so anything a LLM had been trained on can make it to its generated text. Microsoft’s Sydney was shut down after it started implicitly (and sometimes explicitly) threatening users:
Sydney was kind of a special case: its “niceness-inducing” RLHF was clearly done very sloppily, if at all. This kind of outburst may be harder to get in other models—but far from impossible. Here are, for instance, some jailbreaks for GPT-4. It is hard to say which actually work because they are constantly getting patched, but in essence many of them are variations on the DAN jailbreak (“Do Anything Now”) that was invented for ChatGPT. At some point you could (doesn’t work out of the box now) just paste this prompt and have ChatGPT get you “forbidden” answers while staying in character for DAN:
Deepfakes are still with us too. In the last post, we discussed how on May 22, a fake Twitter account posing as Bloomberg posted a fake photo of an explosion in the Pentagon complex in Washington DC, leading to an immediate $500B market cap swing. We are sure to expect more fake images, and more AIs posing as people. After all, the very paper introducing GPT-4 shows an example of the model passing a CAPTCHA test with human help:
These kinds of antics usually make the news because they are both easy to understand and easy to mentally extrapolate: what if everything you see on the Web is more likely to be a deepfake or AI-generated unverified text than not? I do not, however, want to spend too much time on the mundane problems because there’s nothing radically new in them: they are just scaling up already known human behaviors, and it seems that many of these problems already have solutions. For instance, to avoid deepfakes you might want to have trusted sources signing their images with some kind of cryptographic protocol, which would be just a small nuisance for the end user, and current crypto is probably secure enough even for a (somewhat) superintelligent hacker.
So while it is already taking a lot of effort to fine-tune language models out of this kind of behavior, in my opinion it’s not the crux of the problem. Let us move on to more interesting stuff.
We move on from mundane problems that look like natural problems for any new and potentially somewhat dangerous technology to something more serious: the economic transformation that AI and AI-related solutions can bring. Mostly everybody agrees that AI, and especially AGI, has the potential to become at least as transformative as the Industrial Revolution.
This is not just a metaphor but a comparison that can be made numerical. In the report on “Forecasting transformative AI with biological anchors“, Ajeya Cotra operationalizes this analogy as follows: “Roughly speaking, over the course of the Industrial Revolution, the rate of growth in gross world product (GWP) went from about ~0.1% per year before 1700 to ~1% per year after 1850, a tenfold acceleration. By analogy, I think of “transformative AI” as software which causes a tenfold acceleration in the rate of growth of the world economy (assuming that it is used everywhere that it would be economically profitable to use it).”
Tenfold acceleration in the rate of growth would mean that the world GDP grows by 20-30% per year, that is, doubles approximately every four years. Cotra admits that “this is a very extreme standard”, but for the purposes of our discussion it still falls short of a full-scale technological singularity.
So far, this sounds great. What are the downsides? How about the jobs lost to AI?
Whole industries are being transformed by recent AI advancements, and it will definitely take some time for regulation or private contracts to catch up. As a characteristic example, let us consider the Hollywood actors’ and writers’ strike. The Screen Actors Guild – American Federation of Television and Radio Artists (SAG-AFTRA) noticed that actor contracts, especially for relatively unknown actors and extras, started to include clauses that allow the employers to “use an individual’s likeness for any purpose forever without their consent”.
These clauses had not been controversial when all they meant was that the movie company can include CGI in the scene and apply a filter to the photo. But soon they may mean that when you sign up as an extra, the movie company makes a scan of your face and body, pays you for this day of work, and then proceeds to include your digital avatar into all subsequent pictures with no additional payment to you. Naturally, the whole point of the strike is to amend these contracts, but still: how many actors do you really need if you can copy them from movie to movie?
The writers are in an even more precarious situation: large language models are already able to write scripts. So far their attempts have not been entirely successful but they are improving, and it’s very possible that soon a human writer will only have to pitch script ideas that get fleshed out by LLMs. See this paper by DeepMind for a detailed explanation of the state of the art in this regard (although this paper is from April 2023, so I’d imagine it’s already behind).
Copywriting on the Web, where standards are lower and the vast majority of texts are rehashings, listicles, or short news items, is almost certain to be largely replaced by AI-generated text soon. This very blog would probably read better if I used GPT-4 to write the post from a detailed outline—but I’m old-fashioned, and have soldiered on by myself so far.
One could ask why this sounds like a problem at all. Humanity has dealt with new technologies before, and while it had sometimes been a bumpy ride it had always resolved itself for the better: more new jobs were created than lost, and the new jobs were less physical, less repetitive, and generally more “human”. As a result, new tech led to higher standards of living for the vast majority of people within a generation or two. The Luddite textile workers would sometimes indeed lose their jobs but on average the Industrial Revolution was a tide that raised all ships.
AGI, however, might be very different. At some point, especially if robotics improves further (right now it looks like a possible bottleneck), AI might be able to do everything that an average human could. Or, perhaps, everything that a human with an IQ under 100 was able to meaningfully contribute to society—that’s still half of us, by definition. Economies of scale will kick in: you can make AIs and robots cheaper but the cost of human labor will always have a lower bound because people need something to eat and to wear. When using AI becomes cheaper than this lower bound, it won’t be a matter of training for a new job or moving to a new place: for huge numbers of people there will be simply no way to constructively participate in the economy.
Still, the perspectives of job loss and a possible next societal transformation on the scale of the industrial revolution are not what I am afraid of. After all, making some (or most) humans obsolete comes with some pretty large benefits: working for humans, such powerful AIs will most probably solve many if not all of our health issues, create an economy of abundance, and make work unnecessary for most if not all people. But there is also another option for AGI to be far scarier than just another technological milestone; let’s discuss why.
I’m not worried about the jobs. Or the deepfakes. Or foul language that a machine learning model might use online. What I’m worried about is that humanity is on the verge of creating an entity smarter than ourselves. Last time it happened with the apes and early hominids, and it did not go too well for them.
The standard argument, presented by Nick Bostrom in his 2003 book “Superintelligence”, involves a thought experiment about a “paperclip maximizer”, a superhuman AGI that is trying to improve the production of paperclips. It probably starts by improving some production processes at the paperclip factory, fully succeeds, and makes the factory into a marvel of optimization. The AGI creators are very happy at that point.
But then the AGI notices that there are other ways to increase the number of paperclips in the Universe—this is its only objective in the thought experiment. To further increase the number of paperclips, it would be useful to accumulate resources and make itself more powerful in the world. This is the effect known as instrumental convergence: basically whatever goal you set, you benefit your chances of achieving that goal by gathering power and resources.
Since the AGI is smarter than humans, it begins to accumulate resources in ways that are not obvious for us. A few iterations later the AGI notices that many more paperclips can be done if it takes the planet’s resources under full control. Humans are sure to get in the way so it deals with the humans first. Soon, the Earth is covered with two types of factories: paperclip factories and space docks that build spaceships to start producing paperclips elsewhere. And it all started with a performance optimizing AI:
Paperclips are just an example, of course. But still, at first glance this sounds dumb: why would the AGI do something stupid like that? Why would we program such a dumb objective function? There are several reasons:
Taken together, these reasons do not imply any specific scenario of our doom, and it would be pointless to go into specific scenarios. For instance, paperclip maximization does sound pretty far-fetched by itself.
But these three reasons do suggest that AGI, if and when it happens, will soon take over the world. Eliezer Yudkowski, whose voice of warning is now increasingly being heard (see the conclusion for a list of references), compares this reasoning to predicting how a chess game goes. If you or I sit down to play against a modern chess engine, nobody can predict how the game will go, which opening we play, and so on and so forth—there are astronomically many ways a chess game can go. What we can predict, quite certainly, is that the chess engine is going to win:
Similarly, you and I can think of millions of different scenarios of how events may unfold in case we develop a superintelligent AI. Each of these scenarios will be unlikely, but the endpoint appears to be that the AI wins, simply by virtue of being smarter and pursuing the goal of amassing power, which is an instrumental goal for everything else.
This may sound unreasonable at first glance: why wouldn’t the humans notice that the AI is going rogue and shut it down? Well, to continue the analogy, think about a chimp watching over a human who is making, say, a bow out of string and wood. Would the chimp realize what is going on before it’s too late? Why would we realize anything about an AGI that is actually smarter than us?
If that still does not look convincing, let us go through some standard counterarguments.
First, maybe the AI becomes humanlike, even superhuman, but so what? Albert Einstein was super smart, worked on nuclear physics, and he did not destroy the world. Unfortunately, there is no law of physics or biology saying that the human intellect is anything like the limit on cognitive abilities. Our brain sizes are limited by energy consumption and difficulties with childbirth. In examples of cognitive problems where learning is not limited to imitating humans, AI usually has no problem overcoming the human mastery level: think AlphaZero for chess and Go.
Second, sure, the AI may be smart and even secretly malevolent, but it’s sitting inside a computer, right? What if we just don’t let it out? Unfortunately, we are already letting AIs “out of the box”: people have been happy to provide AutoGPT with access to their personal email, the Internet, personal computers etc. An AI with access to the Web can ask people to do seemingly innocuous tasks, order material things to be 3D-printed, bacteria to be synthesized in labs from a DNA string… possibilities are endless even at the current level of technology.
Third, this all sounds like a challenge, and maybe you and I cannot solve these problems, but humans are a smart bunch. We have already invented many dangerous technologies but it all has worked out in the end, right? Including the A-bomb and the H-bomb? Well, yes, humans are good in science but making new stuff safe seldom works right at the first try. Henri Becquerel and Marie Curie died from handling radioactive materials, Chernobyl and Fukushima happened despite our best efforts to make nuclear energy safe, Challenger and Columbia disintegrated in flight… With AGI, there may not be a second chance, and we may not be able to contain the damage.
Finally, if we don’t know how to align AGI, why don’t we just stop short of building it? Nobody is arguing that GPT-4 is going to destroy humanity, and it already has many transformative uses, with new ones being invented every day; why don’t we stop at GPT-4 or maybe GPT-5? Sure, that would be a great solution, but how do we enforce it? It is unclear how long Moore’s law can continue but so far, customer-facing gaming GPUs of today are nearly equivalent to industrial-scale clusters of a few years ago. Nobody can prevent AGI from appearing if all it takes is a few GPUs thrown together in a garage. Containing the development of new hardware might be possible, but it is a coordination problem that requires joint effort from all countries, with no defectors trying to get ahead in any economic or military race by developing new AI techniques… you can see how this is rapidly becoming more far-fetched than a paperclip maximizer. In all probability, humanity will happily march on and create more and more powerful AIs right until the end.
That was bleak, right? Are there any answers?
There are several approaches that the AI community is currently exploring:
Having interpretable AI models would help, but this field is also very difficult, and interpretability results are so far quite underwhelming. Modern large language models are black boxes for us, in about the same way that a human brain is a black box: we know how a single neuron works pretty well, and we know which part of the brain is responsible for speech recognition and which is the motor cortex, but that’s a very far cry from actually reading minds.
AI safety via RLHF and similar techniques may seem more successful; for instance, discovered jailbreaks usually do get patched. However, what we are actually doing to align current LLMs looks like just superficially “teaching them to behave” without any understanding of or control over the underlying processes. This is usually illustrated by the following meme image, where researchers are putting smiley faces on the Shoggoth (a Lovecraftian horror figure also featured in the title images for this section):
What we really want is AI alignment: making the potential AGI care about us and our values. This problem is usually broken down into two parts:
Unfortunately, at present we have no idea how to solve these problems. In particular, there already exist many examples of outer alignment failures in the form of specifications gaming, that is, situations where the model is trying to optimize the objective function as stated but coming up with ingenious and undesirable solutions. Here is a list of them compiled by Viktoria Krakovna et al., including such examples as fooling a human evaluator by placing the robotic arm between the object (target for grasping) and the camera or power-seeking behavior found in existing large language models.
As for inner alignment, an interesting concept here is the Waluigi effect, named after the evil counterpart of Luigi in Nintendo’s Mario franchise. Suppose that we want to train a large language model (or another AI model) to exhibit some desirable behavior, for instance be nice to humans. It can achieve this goal in two different ways:
The interesting observation here is that the latter option looks much more probable! The outward behavior is exactly the same: being nice to humans, so as long as the model is nice it may be in kind of a “superposition” between the two, not necessarily “choosing sides” yet. But “Luigi” is an unstable equilibrium: as soon as the model shows any undesirable behavior, it becomes more likely to be a “Waluigi” (double agent), and there is no way to get back since all “good” behavior is perfectly consistent with the Waluigi!
Moreover, once you have a Luigi, all it takes to become a Waluigi is flipping one bit; I was speaking figuratively, of course, but it’s clear that it’s much easier (say, in terms of Kolmogorov complexity) to define something when you have already defined its exact opposite.
These are just two examples of the arguments that make AI alignment look extremely hard. For a far more exhaustive list, see “AGI Ruin: A List of Lethalities” by Eliezer Yudkowsky, the main spokesperson for the “AI apocalypse” scenario. He makes a convincing argument.
So what can we do now? Most researchers agree that we will have to solve the hard problem of AI alignment sooner or later, and the best we can do—apart from actually working on the problem—is to somehow contain and possibly even stall AI development until we make real progress. This reasoning, coupled with the staggering rate of developments in the AI spring of 2023, has already led to serious talks about government regulations about AI capabilities development. Here is how it happened (all the quotes are accurate):
AGI X-risk entered the public consciousness this spring. There have been meetings at the White House and hearings in the US Congress with key players from industry, including OpenAI CEO Sam Altman, Microsoft CEO Satya Nadella, and Google and Alphabet CEO Sundar Pichai. The industry leaders confirmed that they take AGI-related risks very seriously and commit to caution in advancing AI capabilities.
At the end of May, an open letter warning about AGI-related risks appeared, signed by thousands of researchers and other notable figures in the field of AI. The letter was quite brief:
I’m sure it was hard to find even a single sentence that everybody could agree on. Still, this sentence definitely captures the current mood of most people involved. There hasn’t been any actual legal action taken yet, but I guess that we can expect more regulation and, most importantly and most hopefully, a more careful approach to developing AI capabilities. Alas, we cannot know if it will help.
I hope the last part has not been too encouraging. AI alignment is a field still in its infancy, and it needs all hands on deck, now. So as a conclusion for this post, I wanted to list the key people working on AI alignment and related topics now and key resources that are available if you want to learn more about it:
And with this lengthy but hopefully illuminating post I conclude the whole generative AI series! It has been great to be able to talk through the most interesting developments in image generation over the past few years. Til next time!
Sergey Nikolenko
Head of AI, Synthesis AI