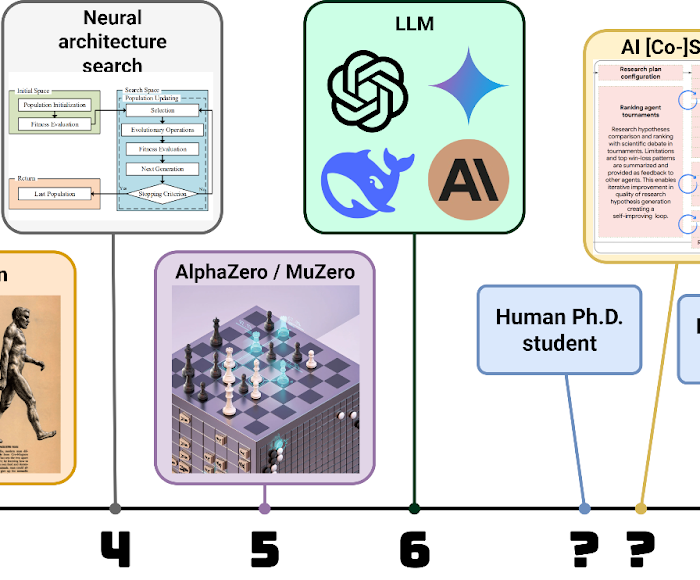
The Creativity Scale: Can AI Do Science?
Today, I want to discuss two recently developed AI...
Facial identification and verification for consumer and security applications.
Activity recognition and threat detection across camera views.
Spatial computing, gesture recognition, and gaze estimation for headsets.
Millions of identities and clothing options to train best-in-class models.
Simulate driver and occupant behavior captured with multi-modal cameras.
Simulate edge cases and rare events to ensure the robust performance of autonomous vehicles.
Together, we’re building the future of computer vision & machine learning
Facial identification and verification for consumer and security applications.
Activity recognition and threat detection across camera views.
Spatial computing, gesture recognition, and gaze estimation for headsets.
Millions of identities and clothing options to train best-in-class models.
Simulate driver and occupant behavior captured with multi-modal cameras.
Simulate edge cases and rare events to ensure the robust performance of autonomous vehicles.
Together, we’re building the future of computer vision & machine learning
Facial identification and verification for consumer and security applications.
Activity recognition and threat detection across camera views.
Spatial computing, gesture recognition, and gaze estimation for headsets.
Millions of identities and clothing options to train best-in-class models.
Simulate driver and occupant behavior captured with multi-modal cameras.
Simulate edge cases and rare events to ensure the robust performance of autonomous vehicles.
Together, we’re building the future of computer vision & machine learning
Facial identification and verification for consumer and security applications.
Activity recognition and threat detection across camera views.
Spatial computing, gesture recognition, and gaze estimation for headsets.
Millions of identities and clothing options to train best-in-class models.
Simulate driver and occupant behavior captured with multi-modal cameras.
Simulate edge cases and rare events to ensure the robust performance of autonomous vehicles.
Together, we’re building the future of computer vision & machine learning
Synthetic data for computer vision to enable more capable and ethical AI.
Exceed Euro NCAP and NHTSA standards.
Car manufacturers, suppliers and AI companies are looking to build computer vision systems to monitor driver state — and occupant activities — and help improve safety. Euro NCAP, NHTSA and others are instituting a range of standards for the development of advanced perception systems, but the high-quality in-car data needed to train AI models is typically low-res and expensive to obtain. Synthetic data can model diverse drivers, occupants, behaviors, and the in-cabin environment to enable the cost-effective and efficient development of more capable CV models in ADAS, DMS and OMS applications.
Model multi-modal camera systems (RGB, NIR) in different in-cabin positions within the car environment. Camera placement and sensor systems are customized for any vehicle’s make and model. Different imaging technologies can be applied to different use cases; e.g., RGB for distracted driving detection, NIR for OMS in low-light conditions.
Vary driver demographics, head pose, emotion, and gestures to model real-world scenarios accurately. Train in-cabin models to comply with Euro NCAP requirements for driver operating status, driver state, driver authentication, eye gaze detection (for both “lizard” and “owl” ocular movement), hands-on-steering-wheel detection, and seatbelt detection.
Meet Euro NCAP standards for driver operating status (talking on phone, eating, etc) and detecting the presence of various activities and objects. Mobile phones and coffee cups are common distractions, and activities that lead to accidents include eating, talking on a handheld device, and in-cabin interactions between driver and occupants.
In addition to automobile security and access, facial recognition can be used to customize a broad range of driver and occupant preferences. Audio and entertainment, seat position, temperature, and other settings can all be configured automatically using computer vision and sensor systems.
The primary objective of an Occupant Monitoring System (OMS) in a car is to enhance safety by providing real-time information about the occupants’ actions and status. The system can detect if occupants are wearing their seat belts, their posture, whether they are drowsy or distracted, and even their emotional state. Synthetic data can model any in-cabin activity, helping car makers meet Euro NCAP requirements for monitoring occupant status, movement, condition, and seatbelts, as well as the presence of pets.
Pedestrian detection systems can alert the driver if a pedestrian is in the vehicle’s path and can even apply the brakes automatically to avoid a collision. Driver-pedestrian interaction models take gaze estimation one step further by providing a deeper understanding of the relationship between a driver and a person outside the vehicle. Euro NCAP’s Vulnerable Road User Protection requirements are designed to improve safety outcomes for pedestrians, drivers and occupants alike.
Precise knowledge of eye gaze direction is critical for DMS models to perform accurately, and is nearly impossible to obtain using low-res, unlabeled real-world training data. Manual annotation of eye gaze direction is particularly susceptible to human error, making synthetic data a compelling option for assessing gaze. Further, understanding and accounting for “owl” and “lizard” eye movement is vital for meeting Euro NCAP requirements.